Query
What are the main risks for corrupt practices in the area of statistics? How can these the risks be mitigated?
Caveat
The extent and forms of corruption that can affect the work of statistical offices depend on a range of variables, including country context, sector, institutional structure and working practices.
Identifying an exhaustive list of corruption risks that could occur in various settings in which statistics are produced is thus not only beyond the scope of this Helpdesk Answer, but also relatively futile. Instead, this paper seeks to provide a framework to understand the various corruption risks that statistical processes may be exposed to and showcase several mitigation measures that could be applied to reduce vulnerabilities.
Background
As noted in the UN Statistical Division’s Fundamental Principles of Official Statistics (2015), “statistics provide an indispensable element in the information system of a democratic society, serving the government, the economy and the public with data about economic, demographic, social and environmental trends”.
Yet forms of corruption in the production and use of statistics can compromise the validity and reliability of the data produced by statistical agencies. The specific corruption risks encountered in a given setting are the function of a range of variables, including country context, sector, institutional structure and working practices (Jenkins 2018).
This Helpdesk Answer aims to present potential risk factors operating at different levels in statistical processes, considers the types of data that may be particularly vulnerable to corrupt manipulation (often as a result of their political sensitivity), and finally discusses potential mitigation measures.
Ensuring integrity in the production of statistics is not only a matter of minimising fiduciary risks and safeguarding public resources from misuse. With the rise of data-driven and evidence-based policymaking, corrupt practices that impinge upon the quality of statistical data can compromise the credibility of public policy. A dearth of reliable statistics can thus have numerous second-order effects with potentially severe implications on citizens (UNSD 2015).
A starting point for policymakers concerned by the potential of corruption to undermine the accuracy of statistical outputs is provided by various statistical standards that set out ethical principles and good practices. Indeed, frameworks developed by international agencies to guide the ethical production of statistics typically cover areas including the independence of statistical agencies, as well as transparency, accountability and impartiality, all of which are highly relevant to efforts to curb corruption in research and statistics (see ASA 2018: 4; UNODC 2018: 34; CSSP 2015: 10).
The real or perceived lack of independence of national statistical agencies, the absence of meritocratic recruitment of the staff, and violations of the principle of impartiality can all threaten the reliability and validity of statistics being produced. As covered below, these are also among the risk factors that can provide opportunities for private gain that corrupt actors can exploit.
Anti-corruption measures in the area of statistics may take various forms, ranging from the traditional emphasis on professional integrity, transparency in methodology and accountability to research subjects, to a more recent focus on creating a culture of open data and communication (Gelman 2018: 40). In short, identifying corruption risks and applying mitigating strategies can help enhance the integrity1862fc97bffd and reliability of statistical outputs as well as enhancing trust in statistical agencies (Gelman 2018: 41; Badiee 2020).
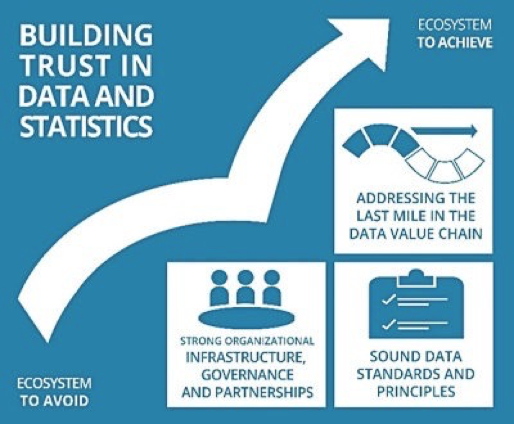
Source: Badiee 2020.
Curbing the pernicious effect that corruption has in undermining the accuracy and impartiality of data can have a potentially transformative impact on sustainable development. As Pali Lehohla, South Africa's Statistician-General, has put it, statistics can act as a “conduit of trust” between citizens and the state, a kind of “currency that holds a global promise for transparency, results, accountability [and…] sustainability” (UNSD 2016). Broad and inclusive participation in the data production process is also key to policymaking relevance and the integrity of the data; Lehohla observes that “the only way statistics can be useful is by engaging actively with the purveyors and politics of policy making and makers, academia, geoscience and locational technology, NGOs, technology, finance, media and citizenry” (UNSD 2016).
International statistical frameworks
Compliance with international principles and frameworks intended to guide the ethical production of statistics can help minimise opportunities for corruption, even if these frameworks are not explicitly designed with anti-corruption in mind. A few examples of international frameworks setting out such ethical principles are covered below.
United Nations Fundamental Principles of Official Statistics and its implementation guidelines
Primarily originating from the Fundamental Principles of Official Statistics put together in 1991 by the Conference of European Statisticians to guide Central European countries to produce appropriate and reliable data that adhered to certain professional and scientific standards, the Fundamental Principles of Official Statistics were later adopted by the United Nations Statistical Commission (UNSD) in 1994 (UNSD 2020). Later, it was updated by the UNSD and subsequently adopted by the UN Economic and Social Council as well as the General Assembly (UNSD 2020).
The 10 principles are as follows (UNGA 2014: 2):
- Recognising the role of official statistics as an integral part of the information system about economic, demographic, social and environmental trends, they ought to be compiled by an impartial agency so that citizens’ entitlement to public information is honoured.
- To sustain trust in the official statistics being prepared by agencies, the methods and procedures for the collection, processing, storage and presentation of statistical data need to be decided on ethical and professional grounds.
- To make sure that data is being interpreted correctly, agencies need to make available the sources and methods of the statistics.
- Statistical agencies ought to have the right to comment on the inaccurate interpretation and misuse of statistics.
- Data for statistical purposes may be extracted from all types of sources including but not limited to surveys and administrative records. The sources need to be chosen keeping factors such as quality, timeliness, costs and the burden on respondents in mind.
- Individual data collected by statistical agencies (for both natural and legal persons) ought to be strictly confidential and used exclusively for statistical purposes.
- The laws, regulations and measures under which the statistical systems operate are to be made public.
- To achieve consistency and efficiency in the statistical system, coordination among statistical agencies within countries is essential.
- National statistical agencies in each country should use concepts, classifications, and methods that promote the consistency and efficiency of statistical systems at all official levels.db90f9f5011d
- To improve statistical systems worldwide, bilateral and multilateral cooperation in statistics ought to be encouraged.
The implementation guidelines for these principles, complied in 2015, are contained in a separate document. These guidelines outline activities that a statistical agency is advised to undertake when trying to develop or implement a particular principle in their respective national contexts. The guide also cites examples from statistical agencies as well as existing national and regional legislative frameworks (UNSD 2015). For example, citing the examples of good practices, the guide mentions the case of the Methodological Council in Slovenia, which was set up to allow external review by academics of the methods being used by the national statistical agency (UNSD 2015: 20).
Recommendation of the OECD Council on Good Statistical Practice
The OECD recommendation toolkit, developed by the OECD Committee on Statistics and Statistical Policy (CSSP), is the only OECD legal instrument with respect to statistics. It serves as a key source for providing detailed plans to establish credible national statistical systems as well as evaluating and benchmarking them (CSSP and OECD Secretariat 2020). In doing so, the toolkit looks at the institutional, legal and resource requirements for statistical systems; the methods and quality of processes of statistical collection, production and dissemination; coordination, cooperation and statistical innovation (CSSP and OECD Secretariat 2020: 6).
The 12 recommendations include (CSSP 2015):
- putting in place a clear legal and institutional framework for official statistics
- ensuring professional independence of national statistical authorities.
- ensuring adequacy of human financial and technical resources
- protecting the privacy of data providers.
- ensuring the right to access administrative sources
- ensuring the impartiality, objectivity and transparency of official statistics
- employing a sound methodology and a commitment to professional standards
- committing to the quality of statistical outputs and processes
- ensuring user-friendly data access and dissemination
- establishing responsibilities for coordination of statistical activities within the national statistics authorities
- committing to international cooperation
- encouraging the exploration of innovative methods as well as new and alternative data sources
International Monetary Fund (IMF) Standards for Data Dissemination
To enhance member country transparency and openness, the IMF has come up with voluntary standards for dissemination of economic and financial data, including (IMF 2019):
- The Special Data Dissemination Standard (SDDS), established in 1996 to guide members that have, or might seek, access to international capital markets in providing their economic and financial data to the public.
- The General Data Dissemination System (GDDS) established in 1997 is for member countries with less developed statistical systems as a framework for evaluating their needs for data improvement and setting priorities.
- The SDDS Plus was created in 2012 as an upper tier of the IMF’s Data Standards Initiatives to help address data gaps identified during the global financial crisis.
- The enhanced GDDS (e-GDDS) replaced the GDDS in 2015.
- Finally, the Data Quality Reference Site (DQRS) aims to develop a common understanding of data quality by defining data quality, describing trade-offs among different aspects of data quality and giving examples of evaluations of data quality.
Currently, more than 97% of IMF member countries apply at least one of these standards.
Other standards
There are regional standards such as the European Statistics Code of Practice (revised 2017), and Code of Good Practice in Statistics for Latin America and the Caribbean. A few examples of legal code being used at the national level include: United States Guidelines for the template for a generic national quality assurance framework (NQAF); UK Code of Practice for Official statistics; and Italian Code of Official Statistics.
Corruption risks in statistical work
There are various ways to understand potential corruption risks in statistical work. The application of any given corruption risk assessment framework to a particular statistical agency would require extensive in-depth research, and thus goes beyond the scope of this answer. Nevertheless, a couple of conceptual models that can help practitioners to identify corruption risks in the work of any given statistical system are covered below.
Data production cycle
A mapping of corruption risks in statistical work could be based on an analysis of a schematic and simplified version of the typical data production cycle. Data processing is simply the conversion of raw data to meaningful information through a given process (PeerXP Team 2017). The main stages of this processing cycle are as follows (PeerXP Team 2017):
- The first stage of the cycle is data collection, which is crucial as the quality of data gathered has an impact on the statistical output. The collection process ought to make sure that the data collected is both defined and accurate, to ensure that subsequent decisions based on the findings are valid.
- Preparation is the manipulation of data into a form suitable for further analysis and processing. Analysing data that has not been assessed for accuracy may produce misleading results.
- Input is the stage where verified data is coded or converted into machine readable form so that it can be processed through an application. In cases of data that have been collected manually, data entry is done through the use of a keyboard, scanner or data entry from an existing source.
- The processing stage is when the data is subjected to various methods of technical manipulations to generate an output or interpretation of the data. This may also be done using machine learning and artificial intelligence algorithms.
- Output/interpretation is the stage where processed information is transmitted and displayed to the user.
- Storage is the last stage in the data processing cycle, where data, and metadata (information about data) are held for future:
In addition to the technical nature of what goes into the process of collecting and converting data into usable statistics, there are other factors that contribute to the production of statistics given that these operations often exist as a part of a broader policy process, and are embedded in a political context. In other words, it is not just about the processing of data – it is about decisions on what to measure, how the data should be interpreted and how the data should be presented to the public.
Taking this into account, the different stages in data production may be roughly understood via the infographic on the following page. Anti-corruption practitioners seeking to assess the potential impact on the work of a statistical agency would do well to consider potential corruption risks arising at each stage.
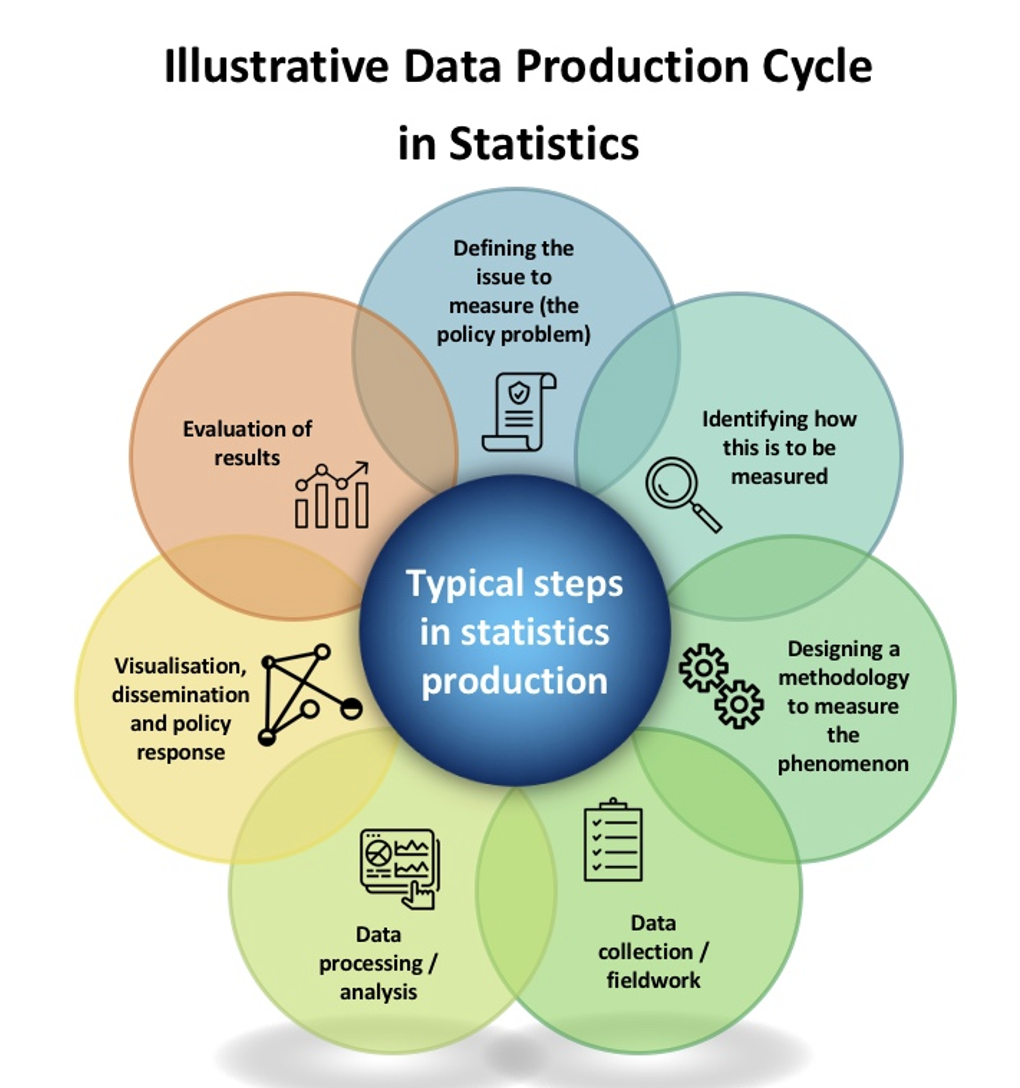
The main steps involved in data production by national statistical agencies may also be understood as depicted in the graphic below.
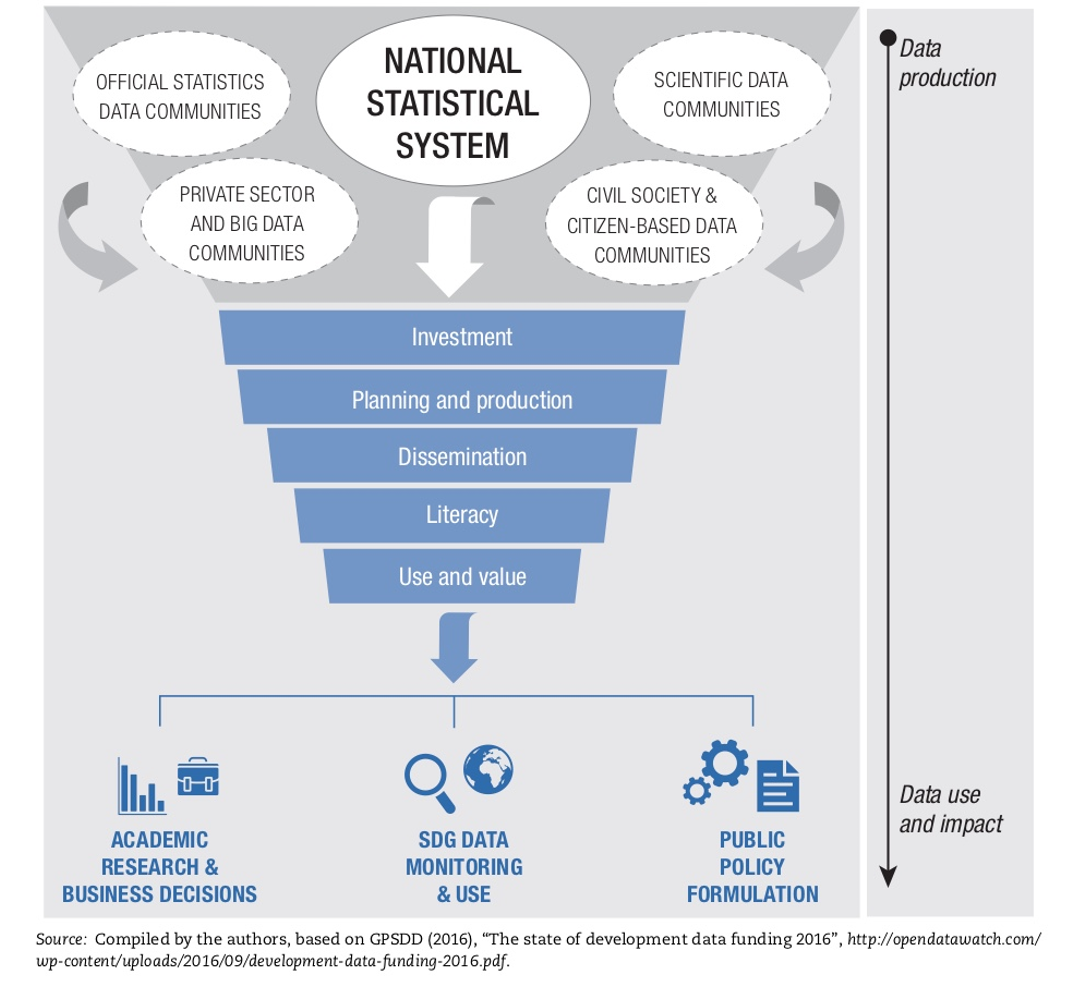
Certain integrity risks are more likely at particular stages of the data production cycle. For example, where a statistics agency is not truly independent, is when: it could be instructed to collect data to embarrass political opponents; it may be prevented from collecting data from those critical of the government; and the government may distort the analysis or prevent the findings from being published. For instance, economists and social scientists have raised concerns over “political interference” in statistical data in India, alleging that any numbers that cast doubt on the government’s achievements seem to get “revised or suppressed” (Economic Times 2019).
Data collection and entry is often time-consuming and is a process that is often outsourced (PeerXP Team 2017). Thus, during the data collection phase, petty bribery and the abuse of per diems could occur by survey enumerators. Similarly, there may be corruption in the process of procuring survey companies. Bribery, kickbacks, bid-rigging and false accounting are a several prominent corruption risks in procurement (OECD 2016).
Value chain analysis
Another method of understanding corruption risks in statistics could be in terms of the level of the value chaine8eea99b6278 at which they occur: contextual, organisational, individual or procedural (adapted from Selinšek 2015 and Transparency International 2017). A few factors that may encourage corruption risks at the different levels are highlighted in the following table (Jenkins 2018, adapted from Selinšek 2015).
Factors encouraging corruption at different levels
Level |
Specific risk factors |
Contextual factors Factors outside of the control of the organisation |
|
Organisational factors Factors within the control of the organisation or sector that are the result of their actions or inactions, such as the rules and policies for good governance, management, decision making, operational guidance and other internal regulations |
|
Individual factors Factors that could motivate individuals to engage in corrupt or unethical behaviour |
|
Working process factors Factors that arise from working procedures in an organisation |
|
Types of corruption risk
Political interference
Since official statistics for economic and social policies can have significant political or financial consequences for a country, this can provide incentives for political manipulation of data (Jenkins 2018). Political advantage as a form of private gain may be a motivating factor for those abusing their power by interfering in the production of statistics. Political manipulation could occur at the policymaking stage of the statistical value chain process and at the design or interpretation phase of the data processing cycle.
Wallace (2015) argues that “some types of data” are more politically sensitive and hence more likely to be manipulated than others, while some periods of time are also more politically sensitive than others (Seltzer 2005), so data reported at these times could be more vulnerable to tampering. For example, it has been noted by scholars that poor economic performance data may be a threat to political administrations, particularly during election periods (Geddes 1999; Wallace 2015; Jenkins 2018). This can provide incentives for the incumbent government to falsify economic statistics (Jenkins 2018). Examples of countries where “election-motivated” data manipulation has occurred include Argentina, Russia, Turkey, Mexico and the United States (Healy and Lenz 2014).
Argentina provides a particularly interesting case study. The country was known for a lack of professional independence of statisticians due to political interference in methods and data, leading to a number of official statistics that were inaccurate. However, efforts to revamp Argentina’s statistical system are currently underway, which include revising the legal framework regulating statistical work. While restoring credibility of official statistics and public confidence in official statistics presents a significant challenge, strengthening independence and ensuring that national statisticians have the exclusive authority to decide on statistical methods and dissemination, as well as focusing on meritocratic recruitment are considered by observers to be potentially effective strategies (CSSP and OECD Secretariat 2020: 19).
Thus, arbitrary political manipulation of concepts, definitions, and the extent and timing of the release of data, doctoring the actual data released, using the agency for political commentary or other political work and politicising agency technical staff could all contribute to political interference in statistical production (Seltzer 2005). Undue influence over the production and presentation of statistics and outright political interference can take a number of forms, including (Aragão and Linsi 2020):
- The executive branch forcing statistical agencies to publish figures that are more favourable to the government than the estimate calculated by technocratic experts.
- In contexts where the level of statistical capacity is low, politicians can leverage this uncertainty about the “actual” value of indicators to their advantage. For instance, a political decision to starve statistical bodies of funds and intentionally keep them weak so they cannot produce data that might embarrass the government.
- Given that international statistical methodological manuals are often adapted by statisticians to suit to local contexts, this can potentially offer opportunities to local officials to interfere with the production of statistics.
- Lastly, indicator management through indirect means, which neither violates the methodology or the data. This includes politicians’ strategies to influence the statistical production process by adjusting operational procedures that are under their control with the intention of tweaking raw data being fed into statistical indicators. Simply put, the statistical agency is directed towards a type/source of data that would in itself support the information outcomes that the political influencer (typically the government) would want.
Self-serving incentives may also guide governments to manipulate statistics to “show progress”. Thus, they may adopt shallow reforms or “quick-fixes” to improve scores on indicators, without addressing the root cause of the governance failings. For instance, law enforcement bodies may be put under pressure to show improvements on the conviction rate, which could – among other issues – lead to speedy trials in which defendants do not receive adequate legal representation (Jenkins et al. 2018).
When it comes to incentives to manipulate administrative data on corruption cases to underreport corruption or to go after political opponents, “state bodies might perceive the data collection as an incentive to either not report such cases, or even suppress opening of cases in order to look good statistically; at the same time, the contrary effect might take place – state bodies might feel the need to show a strong arm and thus increase the number of disciplinary cases, which would only be good, as long as the disciplinary cases are merited” (Hoppe 2014).
Nepotism
Nepotism or clientelism can occur in state bodies where some jobs are reserved for workers that have political or personal connections (Chassamboulli and Gomes 2019). Nepotism may occur at the organisational resourcing level of statistical value chains.
When applied in the recruitment process, such practices of nepotism, political patronage and clientelism may lead to the hiring of individuals who may not be capable, willing or qualified to execute their allocated tasks. Even in cases when they are deemed to be competent to perform their functions, the special preference given to them distorts the principle of fairness, creates unequal opportunities, facilitates corruption, and lowers the efficiency and effectiveness of the organisational performance (Council of Europe 2019). National statistical agencies, as public sector bodies, may also fall prey to such risks. Interviews with practitioners in the field revealed that favouritism can occur in the selection of individuals, not just in hiring but also for training opportunities and workshops abroad.
Counter measures to this include transparency in recruitment processes. Articles 23-24 of the Polish Law on Official Statistics clearly determines procedures of recruitment of the chief statistician (UNSD 2015: 63).
Reputation laundering (whitewashing)
Reputation laundering can occur at the data dissemination phase and at the stage of organisational resources of the value chain. For instance, national governments may donate to or partner with reputable organisations to improve their image abroad (Democracy Digest 2020; Transparency International 2020). Saudi Arabia, for instance, has spent millions of dollars to polish its reputation and suppress criticism from international media on grounds of human rights abuses, using an anti-corruption purge reportedly targeted at opponents (Transparency International 2020). Experiences from practitioners interviewed for this paper suggest that in contexts where statistical agencies are compromised, governments are known to point to partnerships with reputable organisations to use their branding to legitimise incorrect or misleading statistics.
Reputational laundering may also involve perverse incentives minimising or obscuring evidence of corruption, as well as manipulating data to make an agency’s performance appear better than it is (Cooley, Heathershaw & Sharman 2018). In the United Kingdom, in 2013, for example, the chief inspector of the constabulary admitted that manipulation was going on in the recording of crime figures by police officers to show that crime had fallen. There were cases of serious offences, including rape and child sex abuse, which were being recorded as “crime-related incidents” or “no crimes” (Rawlinson 2013). The chief inspector at the time added that while errors in statistics might occur, “the question is the motivation for the errors” and many errors were those of “professional judgment” (Rawlinson 2013).
Per diems abuse
The compensation employees of public and private organisations receive for extra expenses incurred excluding their normal duty is known as per diems, and it can act as an incentive especially in contexts where the normal salary of officials is low (Søreide et al. 2012). Such risks may manifest at the collection stage of the data processing cycle (particularly during labour-intensive activities such as conducting household surveys) and at the organisational resourcing stage of the value chain.
Apart from risks of unnecessary additions to work in a bid to increase per diems, other risks include skimming the per diems of subordinates, or falsifying participation records (Bullen 2014).
Data ownership risks
An infamous example of misuse of data is the Cambridge Analytica case, wherein digital consultants for the Trump 2016 presidential campaign misused millions of Facebook users’ data to build voter profiles (Confessore 2018). On the side of state statistical agencies, there is an increased risk of data loss, theft or misuse through malicious attacks or mismanagement (BSI 2018). There is also a growing threat of shadow information technology (IT) systems, where officials use parallel systems to store and access organisational data without explicit approval (BSI 2018). Data theft and mismanagement can happen at any stage of the processing cycle or value chain. Anecdotal evidence from practitioners interviewed for this paper suggests that individuals inside a statistical agency can monopolise public data, controlling access and even selling this data illicitly to unscrupulous people who can profit from it.
Examples of types of data at risk
Survey data
Such data may be especially subject to the risk of falsification by either the interviewer or the respondent. The American Association for Public Opinion Research (AAPOR) (2003) defines interviewer falsification as the “intentional departure from the designed interviewer guidelines or instructions, which could result in the contamination of the data”. Recently, more attention is being paid to other agents of falsification, including supervisors and other organisations (Stoop et al 2018).
Falsification by the interviewer can comprise (Robin 2018):
- fabricating all or part of an interview
- misreporting and falsifying process data
- recording of a refusal case as ineligible for the sample
- reporting a false contact attempt
- miscoding answers to questions to avoid follow-up questions
- interviewing a non-sampled person in order to reduce effort required to complete an interview
- misrepresenting the data collection process to the survey management
Falsification by the organisation on the other hand consists of (Robins 2018):
- fieldwork supervisor who chooses not to report deviations from the sampling plan by interviewers
- data entry personnel that intentionally record responses incorrectly
- members of the firm itself who add fake observations to the dataset
Incentives for such acts of falsification by the interviewer or organisation may stem from various places. For instance, as discussed in the previous section, per diem abuse is known to be rampant in contexts where salaries are low. Thus, to increase per diems, the number of interviews conducted may be inflated. On the organisational front, there may be pressure from superior authorities to skew results in favour of those wielding power.
Census data
May be manipulated for gerrymandering, which is the practice of drawing electoral district boundaries in a way to advance the interests of the controlling political faction. Gerrymandering is considered a threat to the proper functioning of democracy in a number of countries, including the United States (Guest, Kanayet & Love 2019).
Governance data: such data on governance performance, such as corruption, is often politically sensitive and therefore ripe for misuse. As discussed in the case of misreporting crime statistics by the UK police, such risks of interference as also noted in the production of crime statistics (Harrendorf et al. 2010). The risk of interference is particularly high for corruption surveys, given that the data being produced relates to an illegal activity involving public officials. Indeed, where a national statistics office has insufficient autonomy from the executive, there may be a conflict of interest when it comes to what is essentially a government agency producing data on the probity of public servants (UNODC 2018: 35).
Even where the national statistics body has a high degree of independence, the UNODC’s Manual on Corruption Surveysobserves (2018: 35) that respondents to a survey on corruption conducted by a national statistics office “may disclose their experience in a biased manner, as they feel they are reporting illicit conduct to a government agency rather than as part of a statistical exercise”.
When it comes to producing governance data, therefore, the UNODC (2018: 35) recommends that national statistics offices take a number of steps to “to ensure the quality of the survey, the transparency of their activities and the integrity of their results”. These include the establishment of a national advisory board or technical committee to oversee to the development and implementation of politically sensitive surveys. Such a platform can involve a range of stakeholders to ensure the quality of data produced, including ministries, anti-corruption agencies, academics and civil society representatives. Another approach is to partner with relevant regional and international bodies to draw on their expertise and technical support, as well as to ensure that international best practices, such as the Fundamental Principles of Official Statistics are adhered to (UNODC 2018: 35).
Trade data
Might be manipulated to hide corrupt transactions. Indeed, discrepancies between import and export data can point to grand corruption. The work of Global Financial Integrity (GFI), which analyses publicly available international trade data from the United Nation’s Comtrade database, illustrates how trade mis-invoicing is a persistent challenge that contributes substantially to illicit financial flows around the world.
Another example is the case of Cambodia. In 2016, a US$750 million discrepancy was uncovered between the country’s documented sand exports to Singapore and Singapore’s recorded imports (Willemyns and Dara 2016). Between the years of 2007 and 2015, according to the UN Commodity Trade Statistics Database, the Cambodian authorities reported exporting US $5.5 million worth of sand to Singapore. However, the database shows for that same period, Singapore imported US $752 million in sand from Cambodia. Some observers have pointed to corruption as the source of the discrepancy (Handley 2016).
Macroeconomic data:also discussed under the risk of political interference, macroeconomic data is known to be widely manipulated by governments. In addition to the examples mentioned above, prominent cases include Dilma Rousseff’s attempts to lower Brazilian debt and deficit figures between 2012 and 2015, and controversies about Greece’s public finance statistics in the 2000s (Aragão and Linsi 2020).
Type of data |
Phase of data cycle presenting higher risk of corruption |
Survey data |
Collection and data entry stage (faulty reporting and miscoding of data) |
Census data
|
Collection stage (political interference to skew/bias results) |
Trade data
|
Output/interpretation stage (discrepancy in import and export data) |
Macroeconomic data
|
Processing stage (data may be selectively assessed due to interference) |
Personal data |
Storage stage (data may be sold or misused) |
Governance data |
Data collection and analysis stage (risk of interference and misreporting) |
Developing versus developed contexts
As discussed in the previous sections, manipulating data can be done through various methods. While corruption risks would depend on the situational factors at hand, some methods may be more common in low-income countries, while others may be more prominent in industrialised economies.
For example, political discretion and undue influence over census data collection has been documented in both the US (2020) and Canada (2011). Methodological “sloppiness” in the case of Japan’s monthly labour survey (2019) and methodological controversy in measuring unemployment in France (2007) as well as real and perceived inflation in Italy (2000) are perhaps the result of political sensitivities and interference encountered by statistical authorities in developed settings (Prévost 2019).
Per diem abuse may be more rampant in the Global South where public salaries are usually low. For example, a report prepared by the Public Policy Forum found that in 2009 Tanzania spent US$390 million on per diems, equivalent to 59% of the country’s payroll expenditures (Samb et al. 2020).
Mitigation measures
Independence safeguards
Ensuring the professional independence of statistical authorities is crucial to the life cycle of data production and unsurprisingly a key principle in international manuals on standards for statistical work (UNSD 2015; CSSP and OECD Secretariat 2020).
Measures of independence may also serve as a proxy indicator for the quality of data being produced by a given country (Prévost 2019; OECD Secretariat 2020: 9). (See further details on this subject in discussion of the UK below.) A legislative and regulatory framework providing for the meaningful independence of statistical agencies is a necessary but not sufficient condition for their effectiveness and impartiality. For example, the Bulgarian Statistics Act includes various criteria to ensure professional independence. Specifically, chapter 1 of this act states (UNSD 2015: 108):
- Statistical activity shall be carried out in compliance with the following principles: professional independence, impartiality, objectivity, reliability and cost efficiency.
- Statistical information shall be produced in compliance with the following criteria for quality: adequacy, accuracy, timeliness, punctuality, accessibility and clarity, comparability and logical consistency.
- “Professional independence” is a principle according to which statistical information shall be developed, produced and disseminated regardless of any pressure from political or interested parties.
At the regional level, article 2.1.a: of Regulation (EC) No 223/2009 of the European Parliament and of the Council of 11 March 2009 on European Statistics reads that “professional independence mean(s) that statistics must be developed, produced and disseminated in an independent manner, particularly as regards the selection of techniques, definitions, methodologies and sources to be used, and the timing and content of all forms of dissemination, free from any pressures from political or interest groups or from community or national authorities, without prejudice to institutional settings, such as community or national institutional or budgetary provisions or definitions of statistical needs”.
Simply put, ensuring independence means securing definitions of independence, functional responsibilities of agencies and decisions on the content and timing of statistical releases in legislation and implementation (UNSD 2015).
The UK provides an interesting example of ensuring that statistical work is carried out at “arm’s length” from the executive. The UK statistical system encompasses a number of different statistical agencies:
- UK Statistics Authority (UKSA): oversees the whole statistical system and promotes and safeguards the production of official statistics. It has a statutory objective of “promoting and safeguarding the production and publication of official statistics that serve the public good” (UKSA 2020a).
- Office for National Statistics (ONS): largest independent producer of official statistics in the UK.
- Office for Statistics Regulation (OSR): statutory independent regulator to ensure that statistics are produced and disseminated in the public good.
- Government Statistical Service (GSS): community for all civil servants working in the collection, production and communication of official statistics.
Each of these operate via a strict code of practice and compliance with these practices, which include provisions for independent operations, are supposed to instil “confidence that published government statistics have public value, are high quality, and are produced by people and organisations that are trustworthy” (UKSA 2020b).
Other salient points from the code of practice in the working of systems include directions for staff and senior leaders. Compliance with the code would show that the statistics body in question is (UKSA 2020b):
- ethical and honest in using any data
- has a strong culture of professional analysis
- respects evidence
- open and transparent about the strengths and limitations of its statistics
- communicates accurately, clearly and impartially
- committed to engaging publicly to understand user needs
Compliance also shows that organisations do not (UKSA 2020b).
- share unpublished data and statistics without authorised pre-release access
- selectively quote favourable data
- use other people’s data without checking the data’s reliability first
A survey from 2018 found that 73% of respondents agreed that statistics produced by ONS are free from political interference and 93% agreed that official statistics are important for understanding the UK (Morgan and Cant 2019). Thus, the argument may be made that the presence of and adherence to such good practice measures stemming from the independent operations of statistical agencies may be viewed as acting as a proxy for high quality and trustworthy data.
Culture of openness
A history of opacity in data collection, analysis, and reporting in statistics needs to give way to creating a culture of openness and proactive disclosure (Gelman 2018). Transparency of statistical methods builds trust in the data being disseminated as well as helping in knowledge sharing and capacity building for statisticians (Rivera et al. 2019; Badiee 2020).
Provision 10 of the OECD Recommendation of the Council on Open Government corresponds to an “open state”34ec7c650e86 (OPSI 2020). Such a concept goes beyond open governance and encourages sharing of information and best practices across all levels of government and public institutions to usher in a culture of transparency, integrity, accountability and stakeholder participation.
South Korea, for example, has a national statistics online portal system acting as a “one-stop service” for all official statistics produced by not only Statistics Korea (KOSTAT) but also other agencies that produce statistics (UNSD 2015: 37).
In Mexico, as part of a wider open government strategy to achieve a Digital Mexico. Open data was adopted as an enabler of economic and social growth, a tool to help fight corruption, and a mechanism to promote evidence-based policymaking. The Coordination of National Digital Strategy of the Office of the President of Mexico partnered with the National Institute of Geography and Statistics to set up an Open Data Technical Committee, tasked with aligning national statistical plans with the implementation of the open data policy across the government (OECD 2017a).
According to some observers, harnessing the ongoing data revolution through the adoption of open state approaches has the potential to transform the operations of national statistical systems in rich and poor countries alike (see Badiee et al. 2017).
Transforming information technology (IT) systems
The application of new technology can potentially reduce corruption risks in statistical collection. For, example, the use of computer-assisted personal interview (CAPI) tools, such as tablet computers or other handheld devices, to improve the efficiency and accuracy of census and survey data collection has been applied to contexts such as Uganda’s National Panel Survey in 2011/12; Ethiopia’s Rural Socioeconomic Survey in 2013/14; and South Africa’s Community Survey in 2016 (Badiee et al. 2017).
Using geographic information systems (GIS)-based sampling approaches not only makes surveys accessible to more users but could also act as a verification mechanism to ensure that survey enumerators have actually visited the identified locations (Eckman and Himelein 2019; vMap 2019).
In fact, GIS may also be used to (vMap 2019):
- make a survey location map.
- input survey data results.
- determine the location of targeted households
Practitioners are also using random phone samples16c795d6dcb5 to verify with respondents who survey enumerators claimed to have interviewed to check that they were actually contacted.
Moves to adopt cloud data management systems instead of on-premises servers may also lead to reductions in cyber-security as well as mismanagement threats on an organisation-wide scale (BSI 2018).
Practitioners interviewed for this paper stressed that the main advantage of digitising statistical systems to generate and store data ensures that corrupt individuals are unable to monopolise and control access to data or, even worse, sell it for illicit profit.
Audits
Conducting regular audits not only serves to evaluate tools and protect the security and integrity of statistical databases but also checks if the methods being followed are up to standard (UNSD 2015: 58, 71). Moreover, external audits may be valuable in mitigating corruption risks in donor-funded development projects and which engage with statistical agencies (Merkle 2017).
Improving statistical capacity
Increasing statistical capacity is a long-term process. It involves investing in people and institutions and enhancing environments in which national statistical offices work. A global community of data users and producers, the Partnership in Statistics for Development in the 21st Century (PARIS21), has mapped out lessons for building statistical capacity. These include, but are not limited to, providing leadership training for senior management in national statistical offices, with specific components on change management and leadership; a more demand-led/user-driven focus in the data production process; and a greater focus on the enabling environment, including governance structures (OECD 2017a).
International cooperation
Sharing best practices and lessons learned can encourage the development of a culture of transparency and openness among statistical agencies which, in turn, as discussed in the previous sections, helps in the production of high-quality trustworthy statistics.
To that end, there are several existing knowledge sharing initiatives. PARIS21, for example, works to assist low-income and lower middle-income countries in designing, implementing and monitoring national strategies for the development of statistics (NSDS) and to have nationally owned and produced data for all Sustainable Development Goals (SDG) indicators (PARIS21 2017). Moreover, international cooperation is one of the UN Fundamental Principles of Official Statistics (UNSD 2020).
Increasing statistical literacy
Increasing literacy in the methods and use of statistics may help enhance scrutiny of official statistics, thereby increasing accountability measures from the user end. Finland is active in the field of increasing statistical literacy. Statistics Finland produces a variety of statistical literacy products and engages in awareness creating activities. A few examples are (CSSP and OECD Secretariat 2020: 40):
- production of educational materials such as statistical yearbook for children, periodicals and blogs
- cooperating with universities via statistical literacy competitions
- cooperation with schools (for example: teacher training courses)
- media supported offers (for example: YouTube channel, e-courses, up-to-date information on key social indicators, Facebook services)
- training professional users
- participation in events such as exhibitions, fairs for municipalities or education, visits of statistics Finland by media representatives or members of the parliament
Donor backed initiatives
Through the funding that they offer, donors may play key roles in setting standards for harmonisation, transparency and capacity building of statistical institutions that they engage with (Calleja and Rogerson 2019). Apart from these initiatives, on the funding front itself, the pooling of funding mechanisms to counteract fragmentation caused by development funding and improving funding predictability is one of many steps that development practitioners may take in this regard. Moreover, promoting the transparency of funding activities and results could also contribute to the strengthening of funding instruments for statistical capacity (Calleja and Rogerson 2019).
Other recommendations for development partners include (OECD 2017a):
- contributing to making statistical laws, regulations and standards fit for evolving data needs
- improving the quantity and quality of financing for data
- boosting data literacy and modernising statistical capacity building
- increasing the efficiency and impact of investment in data and capacity building through co-ordinated, country-led approaches
- investing in and using country-led results data to monitor progress made towards the SDGs
- making data on development finance more comprehensive and transparent
Practitioners working with donor agencies and statistical agencies who were interviewed for this paper stressed the importance of long-term engagement. In particular, embedding or seconding advisors for long periods in partner statistics agencies can help donors conduct a meaningful political economy analysis of how statistical bodies in aid-recipient countries operate and relate to the executive branch. The need to build trust between donors and staff in donor-supported statistics agencies, and being able to identify windows of opportunity and allies for reform are likewise considered vital.
- Corruption and integrity are interlinked, as individuals play a role in preventing corruption by acting with personal integrity and making ethical choices (UNODC 2020).
- The International Classification of Crime for Statistical Purposes (ICCS) classifies criminal offences based on internationally agreed concepts, definitions and principles to enhance consistency and international comparability of crime statistics, and improve analytical capabilities at national and international levels espouses categories for corrupt offenses. A detailed report lists definitions for acts of fraud, deception and corruption with categories for disaggregation (UNODC 2015).
- Value chain analysis considers a sector in the context of the processes required to produce and deliver public goods and services. It then analyses the value chain in the sectors of interest at different levels, such as policymaking, organisational resources and client interface. Corruption risks at the policymaking level can include political corruption, undue influence by private firms and interference by other arms of the state. At the level of organisational resources, possible risks include fraud, embezzlement and the development of patronage networks. Lastly, at the client interface, the most common risks relate to bribery and extortion (Jenkins 2018).
- The OECD Recommendation of the Council on Open Government defines the following terms as (2017):
- Open government: a culture of governance that promotes the principles of transparency, integrity, accountability and stakeholder participation in support of democracy and inclusive growth.
- Open state: when the executive, legislature, judiciary, independent public institutions and all levels of government – recognising their respective roles, prerogatives and overall independence according to their existing legal and institutional frameworks – collaborate, exploit synergies, and share good practices and lessons learned among themselves and with other stakeholders to promote transparency, integrity, accountability and stakeholder participation in support of democracy and inclusive growth.
- Random-digit dialling (RDD) is a method of probability sampling that provides a sample of households, families or persons through a random selection of their telephone numbers (Wolter et al. 2009).