The phenomenon of climate finance
Billions of dollars are being invested in interventions to mitigate climate change (CC) or to help adaptation to its consequences. In 2018, global climate finance totalled US$546 billion, of which 59% came from private sources and 41% from public sources. Ninety-two percent (US$500 billion) went to mitigation efforts, 6% (US$34 billion) to adaptation, and 2% (US$12 billion) had dual benefits.a75a553ccab6 In 2017, climate finance (CF) in the form of climate-related bilateral and multilateral overseas development assistance (ODA) was US$68.5 billion, with least developed countries (LDCs) receiving US$13.4 billion or 19.6% (OECD 2019a).
Anti-corruption practitioners need no convincing that a sector worth this much needs corruption controls. Financial losses from corruption are notoriously difficult to determine, but one estimate is that of US$13 billion of multilateral climate funds allocated to the water sector every year, around 7–15% (US$1–2 billion) is lost to corruption.3a7dc0e894fe A significant amount of ODA has established anti-corruption controls that have had a range of success. However, outside of ODA channels, controls around the vast sums of CF are less established, uncoordinated, and not evaluated.
This Brief illustrates the threat posed by corruption to CF, including donors’ exposure to this risk. The key target audience is those in the CC sector who see anti-corruption and governance issues as peripheral to the technical challenges of reducing emissions, yet do not fully realise how corruption can negate their goals.
The key points of the relationship between climate change interventions and corruption are:
- Climate change interventions are worth US$546 billion and have commensurate corruption risks.
- The largest recipients of climate-related ODA are notorious for having significant systemic corruption.
- Corruption undermines mitigation efforts to reduce emissions.
- Corruption decreases the quality of adaptation infrastructure.
- The effectiveness of anti-corruption tools in the climate sector is untested and unknown.
- Past cases of corruption have lessons for future action.
The correlation between vulnerability to CC and perceived corruption is clear, as shown by Figure 1.
Figure 1: Climate change vulnerability and perceived corruption: Rankings of 173 countries from ND GAIN and CPI 2019
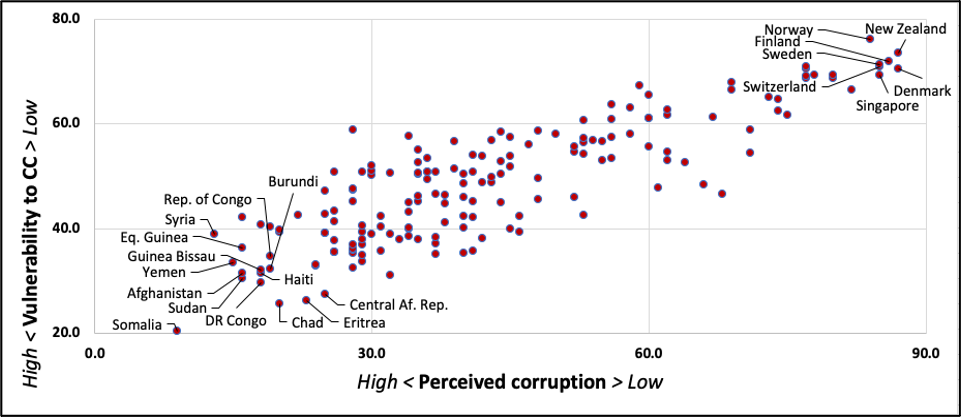
Sources: ND-GAIN 2017 and Transparency International 2019.
Distributing climate finance
Climate finance is ‘local, national or transnational financing – drawn from public, private and alternative sources – that seeks to support mitigation and adaptation actions that will address climate change’.cf66d6cceba2 The UNFCCC’s Paris Agreement categorises CF as either mitigation of carbon emissions – to slow and hopefully reverse CC – or adaptationto the effects of CC, such as improved infrastructure or secure water supplies for vulnerable populations.
Under the UN’s Framework Convention on Climate Change, CF is supposed to be classified as such if it is used for projects that are ‘new and additional’ to ODA.a36905cc650c The implication is that many recipients are getting climate assistance in addition to ODA, expanding the scope for risk around aid and corruption.
The top recipients of CF are among the riskiest places in the world for corruption; the least corrupt recipient is ranked 66 on the Corruption Perceptions Index (CPI) 2019. This is even more the case for the top 10 LDCrecipients: half are ranked in the bottom quintile on the CPI 2019, and the least corrupt LDC in the top 10 is also ranked 66 on the CPI 2019. Yet, the top recipients of CF receive 41.9% of all climate‑related ODA – India alone, a lower middle income country (LMIC), receives 15.1% and the top 10 LDC recipients receive 14.5% (see Table 1).
Total |
28,753,169,000 | – | Total: | 9,914,250,000 | – |
% of all climate ODA |
41.9% | – | % of all climate ODA | 14.5% | – |
All Recipients |
Climate Finance, 2017 | CPI Rank, 2019 | Least Developed Countries | Climate Finance, 2017 | CPI Rank, 2019 |
1. India | 10,320,479,000 | 80 | 1. Bangladesh | 3,742,380,000 | 146 |
2. Bangladesh | 3,742,380,000 | 146 | 2. Uganda | 1,428,141,000 | 137 |
3. Indonesia | 2,711,054,000 | 85 | 3. Ethiopia | 1,404,063,000 | 96 |
4. China | 2,442,507,000 | 80 | 4. Mozambique | 581,233,000 | 146 |
5. Brazil | 2,368,243,000 | 106 | 5. Myanmar | 567,466,000 | 130 |
6. Morocco | 1,637,579,000 | 80 | 6. Cambodia | 560,671,000 | 162 |
7. Mexico | 1,434,938,000 | 130 | 7. Afghanistan | 442,106,000 | 173 |
8. Uganda | 1,428,141,000 | 137 | 8. Tanzania | 412,139,000 | 96 |
9. Ethiopia | 1,404,063,000 | 96 | 9. Senegal | 409,119,000 | 66 |
10. Argentina |
1,263,785,000 | 66 | 10. Nepal | 366,932,000 | 146 |
* lower middle income countries
** upper middle income countries
Sources: OECD 2019a and Transparency International 2019.
Table 2 shows that 89% of CF is concentrated in three sectors: renewable energy generation (60%), low-carbon transport (23%), and energy efficiency measures (6%) – all focused exclusively on mitigation. The remaining 11% of CF is spread over 11 other sectors. Within the 6.3% of CF focused on adaptation, three sectors absorb 78.3% of all funding: water and wastewater management (31.9%), disaster risk management (26.1%), and natural resources management (20.3%).
Table 2: Global climate finance by sector, 2018
Total |
34.5 | 100.1 | 500.2 | 100.1 | 11.9 | 546.6 | 100.66 |
As a % of all CF |
6.3% | – | 91.5% | 2.2% | 100% | ||
Sector |
Adaptation | Mitigation |
Dual Benefits |
Combined |
|||
US$bn |
% |
US$bn | % | US$bn | US$bn | % | |
Disaster risk management | 9.0 | 26.1 | 9.0 |
1.68 |
|||
Coastal protection | 0.1 | 0.3 | 0.1 |
0.02 |
|||
Manufacturing/industry/extractives | 0.1 | 0.3 | 0.1 |
0.02 |
|||
Infrastructure/built environment | 3.0 | 8.7 | 3.0 |
0.56 |
|||
Natural resources management* | 7.0 | 20.3 | 9.0 |
1.8 |
16.0 |
2.99 |
|
Water and wastewater management | 11.0 | 31.9 | 3.0 |
0.6 |
14.0 |
2.62 |
|
Policy and capacity building | 0.3 | 0.9 | 0.3 |
0.1 |
0.6 |
0.11 |
|
Others/cross-sectoral | 4.0 | 11.6 | 8.0 | 1.6 | 12.0 | 2.24 | |
Energy efficiency | 32.0 | 6.4 | 32.0 | 5.98 | |||
Low-carbon technologies |
0.4 | 0.1 | 0.4 | 0.75 | |||
Non-energy GHG** reductions |
0.5 |
0.1 | 0.5 |
0.09 |
|||
Renewable energy generation |
322.0 |
64.4 |
322.0 |
60.22 |
|||
Low-carbon transport |
122.0 |
24.4 |
122.0 |
22.82 |
|||
Energy transmission/distribution systems |
3.0 |
0.6 |
3.0 |
0.56 |
* Includes agriculture, forestry, land use, and natural resources management.
** greenhouse gas
Source: Buchner et al. 2019, p.30.
The concentration of CF in certain sectors is a red flag given these sectors often involve very large projects. For example, the Lake Turkana Wind Power Project is the largest private investment project in Kenya’s history and the biggest single windfarm in Africa,b2f80aa44835 and the Grand Ethiopian Renaissance Dam will be the largest hydroelectric generator in Africa and will double Ethiopia’s power supply.1cf30b5c1fea The scale of these renewable energy projects creates risks for corruption commensurate with their large size.
There are inherent risks for corruption in CC interventions, including:
- Large amounts of money
- Unclear and evolving rules
- Complex institutions and disbursement mechanisms
- Inadequate monitoring
- Highly technical science that is not well understood by others
- Endemic corruption in key sectors, eg construction, energy, and forestry
- Multiple actors with varying anti-corruption controls
- A spending imperative due to urgency (the climate crisis) that can detract from illegal behaviour
Notwithstanding the potential role of these risk factors in undermining CC interventions, analyses of success or failure frequently omit any substantive discussion – or any discussion at all – of governance issues (see for example: Environmental Defense Fund,761debb67e30 Cames et al.,75df72b6a99e Kollmuss et al.,49ab1001247a and SIDAa653caa626fb). A key reason for this absence is the limited research funding allocated to social science aspects of CC, such as corruption.49ea44c2fe35 The lack of research means corruption risks within CC remain poorly mapped and anti-corruption tools undeveloped, untested, and inadequately evaluated.
The impact of corruption
Emissions levels are the result of complex interactions between multiple variables, including income, urbanisation, industrialisation, forestry policy, energy policy, and the quality of environmental governance.e768171286e9 Climate change interventions can work in tandem with industrial, forestry, and energy policies to reduce emissions, but optimising them means better understanding and managing of their risks for corruption.
Corruption in CF has two basic negative impacts on CC interventions:
- Mitigation measures do not reduce carbon emissions as desired, or even cause an increase.
- Adaptation measures will be suboptimal.
While the former affects everyone, the latter affects targeted beneficiaries. Donors and other funders suffer the loss or misuse of funds in both cases.
More analysis is needed to understand the precise role of corruption in CF, but its potential impact on emissions is clear. Corrupt acts (eg bribery, illegal gifts, kickbacks, unmanaged conflicts of interest, or lobbying) can:
- Weaken the quality of environmental regulations so that emissions standards are reduced3a72f1f4ca7c
- Reduce the effectiveness of clean energy programmes, and thus their impact on emissions, by channelling funds into less deserving projects – or no projects0641bcd35f92
- Increase rates of deforestation by encouraging authorities to ignore illegal tree-cutting, causing less carbon to be captured04f1f22b9c5e
- Prolong or promote investments in non-renewable energy, increasing emissions and causing failure to achieve reductions targetsafd56db82e01
- Enable corporate interests to ‘capture’ individual politicians or the state generally through lobbying, thereby shaping government policy away from climate actionfe3d25d2e096
Lobbying is particularly contentious. Occurring in both democracies and non-democracies, it is frequently poorly regulated or falls outside legal definitions of corruption. Lobbying by global corporations at CC discussions, such as over emissions targets, is a frequent target of critics.db925793c632
The impact of corruption on adaptation interventions is more direct and easier to identify, because it is similar to other ODA-funded activities where risks are known:
- Fraud and theft reduce the funds available for activities such as adaptive infrastructure, water supply, soil conservation, or developing resistant crops.
- Bribery and unmanaged conflicts of interest cause biased allocation of funding or location of activities, which means adaptation measures are suboptimal because the best supplier or vulnerable community is omitted.
- Corrupt influences weaken regulatory enforcement of adaptive programmes.
Some CC interventions already incorporate anti-corruption tools, such as the UN’s Reducing Emissions from Deforestation and Forest Degradation (REDD+) programme. However, in global terms REDD+ is tiny. In 2017 the funds disbursed for REDD+ totalled US$19.3 million,4dc47b91f781 compared to US$500 billion for mitigation (see Table 2). REDD+ also has declining importance, as other mechanisms attract more investment. Although anti-corruption lessons from REDD+ are relevant to similar payments-for-ecosystem-services schemes, they lack relevance for renewable energy, low-carbon transport, and energy efficiency – the sectors that account for 89% of CF. Research into how to prevent corruption in these three sectors is what is most urgent.
Case studies of corrupted climate action
Case studies of corrupted interventions demonstrate how climate action goals can be undermined, and highlight lessons for CF-funded action. Unfortunately, cases that can explicitly link failure of interventions to corruption are not easy to identify because evidence is difficult to obtain (eg lobbying leading to illegal financing) or because legal details are not disclosed.
Bribery in forest carbon capture
REDD+ involves 65 partner countries. In Indonesia, three REDD+ projects that were supposed to conserve forests suffered from illegal logging, artisanal mining, and palm oil planting. Villagers reported that private companies paid bribes to local government authorities and police in return for ignoring these activities.a2025b239dc7 The total surface area affected was approximately 1,700 km2 (just a little smaller than Mauritius).
Impact and lessons learned
Rather than cause a loss to project budgets (two projects were partially funded by the Danish and German governments), the primary impact was on emissions. The corruption reduced anticipated carbon capture by about 15%, which was the percentage of area degraded by illegal activities. The lesson is that systemic weaknesses – such as lack of monitoring or reporting mechanisms – need to be identified and reformed, and the perpetrators charged.
Fraud in renewables
Following a new Italian government policy to promote renewable energy through the construction of windfarms, the Mafia targeted the sector. Three individuals, including a former provincial governor, conspired to control a committee and demanded kickbacks to allocate licences and construction contracts for windfarms. Police charged the three men with conspiracy to defraud, bribery, and favouritism.34cd17bba9b2
Impact and lessons learned
Windfarm development became driven by personal enrichment rather than increasing clean energy. The impact on emissions is unclear, but some turbines were located in suboptimal (less windy) areas. One man received a seven-year jail sentence; the former governor received a two-year sentence for abuse of office. This case shows how a renewables policy and CF budget in any country can be subverted by corrupt networks if oversight is weak.
Kickbacks in geothermal energy
In 2008, as part of its renewable energy policy, Kenya created a state‑owned Geothermal Development Company which was 80% funded by donors, including the African Development Bank. The company was plagued by allegations of corruption and mismanagement, and its first project took ten years to implement. A whistleblower reported that the CEO, General Manager, and five tender committee members inflated a contract to move equipment by US$19.5 million, when the winning supplier had previously charged another client US$230,000 for similar work. The allegation was that the perpetrators intended to share the kickbacks from the company (the US$19 million excess).4e7ef4f2ab61
Impact and lessons learned
Climate financeloans were mostly misused or stolen, meaning the company neither reduced emissions nor generated power for energy-poor Kenyans. The court case is ongoing but currently postponed. Competent ethical management, backed up by anti-corruption safeguards, is critical if clean energy businesses are to reduce emissions, generate electricity, and use CF as planned.
Bribery in adaptation infrastructure
Jakarta is subject to increasingly catastrophic flooding because of subsidence caused by excessive extraction of ground water and rising sea levels (40% of the city lies below sea level). To better manage incoming sea water, the Indonesian government is building a US$40 billion ‘giant dyke’ comprising 17 new islands in Jakarta Bay (the design phase was funded by Netherlands). The director of the company developing one island bribed a city councillor with US$148,000 to bypass planning regulations. The entire project was halted in 2016 due to environmental, social, and corruption concerns. The company director was jailed for three years, and the city councillor for ten.48976c819c33
Impact and lessons learned
The halting of the project has delayed flood protection for millions of people. The intrinsic quality of the construction is also unclear: will the infrastructure, built by the company that paid bribes to get the contract, stop the rising sea water? This case demonstrates that adaptation projects have corruption risks like other projects, especially around contracting.
Implications for overseas development assistance
Vulnerability to climate change and risks for corruption are creating a ‘perfect storm’ of governance problems for a subset of developing countries, which have significant needs because of both poverty and weak capacity to initiate or manage mitigation or adaptation activities. Implicated in this situation are donors. (Corporate sources of CF are less exposed because they invest less in such countries.)
Figure 2 shows the top 10 most exposed members of OECD’s Development Assistance Committee (DAC) in terms of the percentage of ODA they direct to the countries ‘most vulnerable’f61fe6755d63 to CC. The presence of relatively small donors is due to the concentration of their ODA on key vulnerable countries: the Sahel for Luxembourg; Papua New Guinea for Australia; Ethiopia and Democratic Republic of Congo for Ireland; and D.R. Congo for Belgium. (Asterisked donors are U4 partners.)
Figure 2: Top 10 DAC members: Exposure to climate change-vulnerable recipients, 2017

Source: OECD 2019b.
Figure 3 shows DAC members whose general ODA is exposed to 11 countries that are both most vulnerable to CC and perceived to be highly corrupt.3d8b3d0569b8 Most of this exposure is due to ODA to Afghanistan, D.R. Congo, Somalia, or Yemen. The good news for donors is that they send only 7–13% of ODA to these recipients, so 87–93% of ODA does not go there. The bad news is that these recipients are home to 270 million people and have extreme humanitarian and development needs that are not being met.
Figure 3: Top 10 DAC members: Exposure to climate change-vulnerable and highly corrupt countries, 2017

Source: Compiled by authors from CPI 2019, ND-GAIN 2017, and OECD 2018.
Going beyond technical issues
Climate finance has a corruption problem. Much of CF is disbursed in highly risky environments and, therefore, optimising CF is not just a question of good technical design to reduce emissions or narrowly monitoring ‘carbon accounting.’ To maximise the impact and effectiveness of adaptation and mitigation interventions, strategies are needed to ensure CF is not stolen, wasted, or directed to suboptimal activities – all problems caused by corruption.
If global warming is to be contained to 1.5°C above pre-industrial levels – the level at which the most catastrophic consequences of climate change can be avoided – there is a 10- to 30-year window to reduce emissions.ce9a9e2c5efa Some countries have a more important role in achieving this than others: large industrial countries such as China, US, India, and Russia can cut their emissions, but other countries have great potential to capture carbon through their forests, eg Brazil, D.R. Congo, Indonesia, and Peru. This second group needs CF to preserve and manage their forests but corruption threatens their ability to mobilise it as intended.
Even with a rise in temperatures of 1.5°C, billions of people will still need adaptation interventions to avoid the worst consequences. This means CF has to be optimised for impact – an issue for climate change practitioners, and for donors and other stakeholders who provide such finance.
Anti-corruption tools give CC practitioners a better chance of limiting global warming to 1.5°C, eg transparency in policymaking; accountability in decision-making; ‘bottom-up’ engagement with climate-affected communities and civil society; controls around fraud, bribery, and procurement; regulation of lobbying; and financial monitoring. Some CC interventions already use these and other anti-corruption measures, but we lack a comprehensive understanding of what these are, the corruption risks they target and, most importantly, if they work.
Finally, while smaller CC sectors should not be ignored, the thrust of anti-corruption efforts needs to focus on renewable energy, low-carbon transport, and energy efficiency, because these are ‘where the money is.’ There is, however, little research on how corruption undermines these sectors’ climate goals.
- Buchner et al. 2019.
- GIZ 2019.
- UNFCCC 2020.
- UNFCC 1992; article 4, paragraph 3.
- Court 2015.
- The Economist 2020.
- 2017.
- 2016.
- 2015.
- 2019.
- Overland & Sovacool 2020.
- See Dash et al. (2020) for a discussion of these factors’ interaction in 61 developing countries, 1990 to 2015.
- Wang et al. 2018; Fredriksson et al. 2004.
- Gennaioli & Tavoni 2016; Kakah 2020; Muchira 2018.
- Enrici & Hubacek 2018; Koyuncu & Yilmaz 2009.
- Transparency International Bangladesh 2019; Project 90 by 2030 2017.
- Gecko Project 2018.
- Taylor & Harvey 2020; Boehmer 2019; InfluenceMap 2019; Fagan-Watson 2015; Wolford 2015.
- UNFCCC 2018; 80.
- Enrici & Hubacek 2018.
- Gennaioli & Tavoni 2016; La Repubblica 2010.
- Kakah 2020; Muchira 2018.
- Gokkon 2017.
- ‘Most vulnerable’ is defined as a rank of ≤160 on University of Notre Dame’s Global Adaptation Index (ND-GAIN 2017), equating to a score of ≤35 (Burkina Faso and Papua New Guinea are equal 161st; thus there are 21 countries). A low score indicates greater vulnerability.
- Eleven countries are in this subset, defined as having a ND-GAIN ranking of ≤160 and a CPI 2019 ranking of ≤160: Somalia, Chad, Eritrea, D.R. Congo, Sudan, Haiti, Afghanistan, Guinea Bissau, Burundi, Yemen, and Republic of Congo.
- IPCC 2018.